Large language models like ChatGPT can help us to better understand the connection between language and thought. This is an opportunity for a new enlightenment.
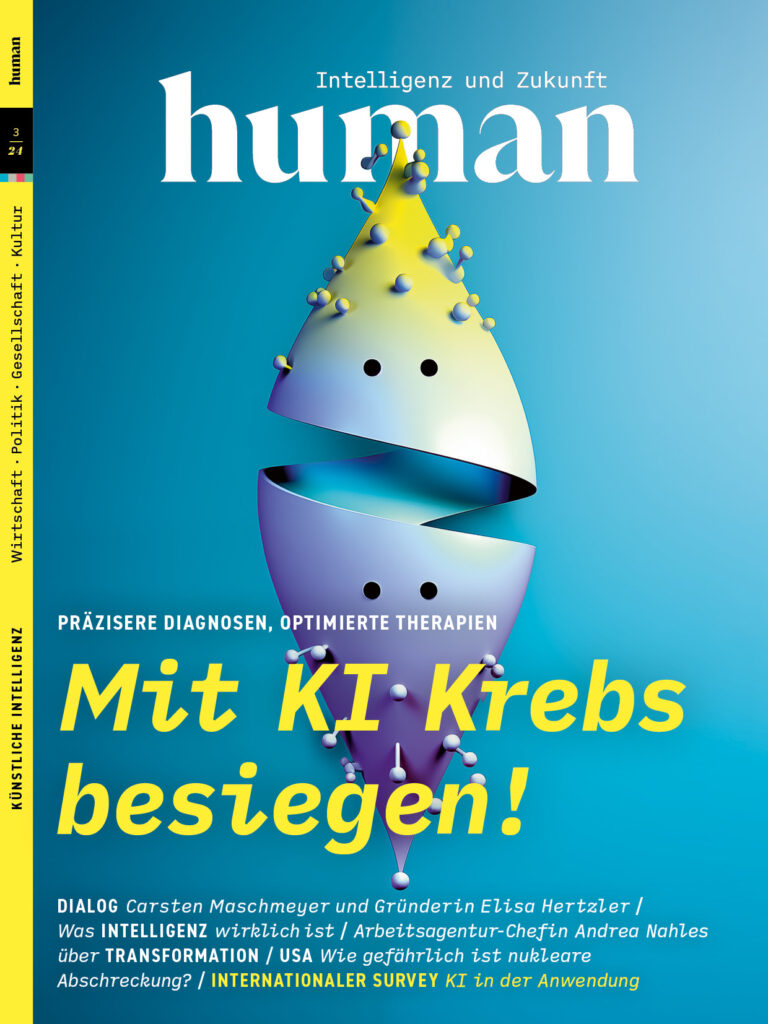
/****
A slightly abridged version of this text appeared in the German magazine “Human” 3/24. The issue can be ordered. [German Version]
****/
Since its invention in the course of the Dartmouth Workshop of 1956, the term “artificial intelligence” has been the subject of a battle of interpretation that its users ultimately always lose. Artificial intelligence research is to intelligence what negative theology is to God. It constantly finds out what intelligence is not. We learned early on that mental arithmetic of all things is the simplest problem to solve digitally, that even sorting apparently doesn’t require much intelligence, that not even playing chess or Go is a definitive proof of intelligence, that cats can be distinguished from dogs or even driving a car apparently can be done without a great deal of intelligence.
In the face of the current hype surrounding generative artificial intelligence – image generators like Midjourney or large language models (LLMs) like ChatGPT – the question arises again: is the astonishingly correct use of words and images by machines “intelligent”?
One faction believes to recognize a “spark of general intelligence” in the large frontier models such as GPT-4, Claude 3, Gemini 1.5, the other faction believes that we are only dealing with “stochastic parrots”, a kind of autocorrection on speed. So the dispute is about “cognition” and whether the “intelligence” is in the machine. It seems more sensible to me to first clarify the relationship between language and thought.
Derrida and the Linguistic Turn
In the second half of the 20th century, the “linguistic turn” occurred in the Humanities. Roughly speaking, the assumption that the possibility of thought is linked to the use of language became widespread, a thesis that is still discussed today in cognitive science as the Sapir–Whorf hypothesis. According to this thesis, we have no direct access to the world because our perception is already symbolically mediated. The cultural studies theories that emerged at the time, in particular “structuralism”, thus sought to make the hidden structural influences of language on thought visible.
Jaques Derrida, as a representative of “post-structuralism”, went one step further and showed that even the signs themselves have no direct reference to the world. Language is not a gateway to reality, but a free-floating system of symbolic referentiality. Derrida’s texts are difficult to understand, but to illustrate his point for our purposes, it is enough to pick up a dictionary. If you look up a word, you will only ever be referred to other words, and if you look them up, you will only find more words, etc. According to Derrida’s thinking, signs only ever refer to other signs, rather than to some kind of “reality”.
The mere fact that LLMs can spit out semantically correct sentences based only on linguistic utterances, without any reference to reality, seems to fundamentally confirm this thesis. However, the closer you look at the technology of AI, the more you get the impression that large language models are operationalized post-structuralism.
Meaning in latent space
Large Language Models always output the next word using probabilistic calculations. In contrast to traditional autocorrect, the LLM not only includes the previous word in the probability calculation, but all previous words. And these previous words are not simply included in the calculation as a combination of letters, but as so-called “embeddings”.
Words or parts of words are called “embeddings” if they are put into a relationship to all other words within a „vector space“. This vector space in LLMs is also called “latent space” and can be imagined as a thousand-dimensional network of terms and all their occuring relationships. The latent space is the result of the LLM’s basic training, in which all the different ways in which terms can be related to each other were stored by statistically measuring through millions of texts.
Since all the connections are precisely weighted, the expanses of this highly complex network cloud contain both close and distant relationships of all kinds: functional, syntactical, legal, foreign-language, ethical, political, aesthetic, etymological and, of course, numerous associative constellations. The Latent Space is a rugged, multi-dimensional landscape of our language.
If we zoom into this network, we find, for example, the word “king”, which has a specific location in this network that results from the connections to thousands of other words. One of these vectors, with which “king” is associated, is the vector “man”. If you subtract “man” from the “king” vector and add the “woman” vector, you end up in the latent space with the word “queen”.
On closer inspection, the latent space turns out to be a more complex variant of Derrida’s dictionary. And just as the dictionary promises us orientation in terms, the latent space of the LLM serves as a map of language. And just as the road network maps out all possibilities for getting from A to B, in Latent-Space all existing and possible sentences, paragraphs, essays or books are laid out as latent routes.
For Derrida, meaning is an effect of moving within this network. It manifests itself in reading, speaking, writing, and thinking as a concrete route from one point in the network to another. Reading, speaking, writing, and thinking are thus navigational maneuvers within this bizarre landscape, in which not all paths are equally probable. Those who want to be understood follow the well-trodden paths.
Technically, you can imagine the process like this: when reading the prompt, the model follows the predefined path (the prompt) within the latent space, word for word, enriching what it reads with all kinds of “embedded”, i.e. multi-dimensional contextual semantics. At the end of the prompt, it then turns its position in the network into the starting point for an independent navigation, the aim of which is to extend the given path to its conclusion in a “plausible way”.
To put some distance between LLMs and humans again, it helps to imagine the latent space of LLMs as a limited and reduced dimensional “impression” of human semantics. Just as the footprint does not represent the whole foot, the latent space also lacks a number of dimensions that we humans include in our references when reading, speaking, writing, and thinking. Emotional, social, material, and even cognitive vectors of consciousness are simply not available to the LLM. You could say that machine semantics is broad and flat, while human semantics is deep and narrow.
Semantics all the way down
But what does this tell us about the machine’s ability to think? It means, first of all, that part of what we perceive as intelligent in humans, and more recently in machines, lies outside the brain and the data center. A good deal of human intelligence is encoded in language, in shared semantics. And this is not primarily a technical or cognitive discovery, but rather one that impacts cultural studies.
This becomes clear if, with Niklas Luhmann, we understand “semantics” as the “stock of meaning of a society”. It is not just about language and writing, but about all conceivable forms of meaning. Although image and audiovisual semantics are also made operational by the image, audio and video generators, we have to imagine the human semantic space as much more comprehensive. From “indicating left turn” to history, from the middle finger to the scientific experiment, from dark metal to the spring collection. The way I move my hand is semantics, “zeitgeist” is a very specific set of semantics, a single look can overflow with semantics, every couple develops an intimate private semantics, even grammar is a semantics and what a dog experiences when it walks through the forest, surrounded by millions of exciting smells, is a thicket of semantics that are plausible to him.
When Heidegger speaks of language as “the house of being”, he means our inclusion in this network of semantics. Each of us inhabits only a small part of this overall structure, and this part essentially determines what we are able to think at all. We are born into our semantic section and have been working ever since to expand it, looking for connections, learning words, works and gestures, and some rooms we have not entered for quite some time.
From world model to program
In a sense, the post-structuralist view thus seconds the notion of the stochastic parrot, albeit with the addition that human thought also consists to a large extent of stochastic semantics routing.
The opposing side always points to the “reasoning” abilities of models such as GPT-4 or Claude 3, and indeed it is astonishing how they can not only produce semantically correct sentences like “The ice melts in the sun,” but also perform surprisingly well in exam papers and other benchmarks. LLMs show themselves to be surprisingly empathetic and creative and can apply theories and methods correctly in a wide variety of contexts. The developers of the systems beliefe that the LLMs have developed a “world model” in the course of their training that allows them to use these often imperfect “reasoning” abilities.
We now have a simpler explanation: no one doubts that language is a system of rules at the orthographic and grammatical level, and LLM shows that this also applies to meaning and to all concepts, logics, methods and theories. Whether grammar, algebra, multistakeholder analysis or interpreting poetry: these are all rule-based thought templates, patterns of correct expression or factories of probable sentences.
Francois Chollet, an AI researcher and Google employee, calls these macro-semantic rule complexes “programs”. Of course not in the literal sense of machine-readable code, but rather as macro-semantic paths that have sedimented in the learning process and were generalized for applicability. Just as plausible words are strung together when formulating sentences, statements are arranged along predetermined paths when macro-semantic programs are applied. By applying them, LLMs work their way through the corresponding context and perform their rule-based operations on it, in order to generate an expected output.
We humans have also practiced many of these macro-semantic programs, sometimes consciously, but more often unconsciously. And because they also determine our view of the world, I see an emancipatory mission resulting from the invention of the LLM. This archive is incredibly deep and possibly contains the programs for all our thinking. Extracting, examining and debating these social semantics of ours, offers the possibility of a new enlightenment.
Pingback: Dickicht der Bedeutungen | ctrl+verlust
Pingback: Krasse Links No 28 | H I E R